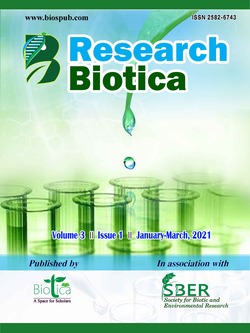
Application of Ground-Based Remote Sensing in Identifying Biotic Stress: A Review
Jitendra Kumar*
ICAR-Indian Institute of Soil Science, Nabibagh, Bhopal, Madhya Pradesh (462 038), India
Ananta Vashisth
ICAR-Indian Agricultural Research Institute, Pusa, New Delhi, Delhi (110 012), India
Nishant K. Sinha
ICAR-Indian Institute of Soil Science, Nabibagh, Bhopal, Madhya Pradesh (462 038), India
M. Mohanty
ICAR-Indian Institute of Soil Science, Nabibagh, Bhopal, Madhya Pradesh (462 038), India
Alka Rani
ICAR-Indian Institute of Soil Science, Nabibagh, Bhopal, Madhya Pradesh (462 038), India
R.S. Chaudhary
ICAR-Indian Institute of Soil Science, Nabibagh, Bhopal, Madhya Pradesh (462 038), India
DOI: https://doi.org/10.54083/ResBio/3.1.2021.28-32
Keywords: Biophysical attributes, Biotic stress, Hyperspectral Remote sensing, Satellite
Abstract
The remote sensing technique has been used for diverse applications in agriculture. An array of continuous narrow wavebands in the hyperspectral remote sensing provide an understanding of the subtle changes in biochemical and biophysical attributes of crops and their different physiological processes. Hyperspectral remote sensing has also been used in discrimination of crops and their cultivars, assessing abiotic and biotic stresses, quantitative estimation of crop nutrient status and soil health. Knowledge of biotic and abiotic conditions over large areas bears the potential to reduce agricultural losses in terms of productivity. Therefore, this article aims to present an overview of the quantification of different biotic and abiotic stress by remote sensing techniques and focuses on future directions for researchers.
Downloads
not found
Reference
Ausmus, B.S., Hilty, J.W., 1971. Reflectance studies of healthy, maize dwarf mosaic virus-infected, and Helminthosporium maydis-infected corn leaves. Remote Sensing of Environment 2, 77-81.
Baret, F., Houlès, V., Guerif, M., 2007. Quantification of plant stress using remote sensing observations and crop models: The case of nitrogen management. Journal of Experimental Botany 58(4), 869-880.
Blackburn, G.A., 1998. Spectral indices for estimating photosynthetic pigment concentrations: A test using senescent tree leaves. International Journal of Remote Sensing 19(4), 657-675.
Clark, R.N., King, T.V.V., Ager, C., Swayze, G.A., 1995. Initial vegetation species and senescence/stress indicator mapping in the San Luis Valley, Colorado using imaging spectrometer data. In: JPL, Summaries of the Fifth Annual JPL Airborne Earth Science Workshop. Volume 1: AVIRIS Workshop. pp. 35-38.
Colwell, R., 1956. Determining the prevalence of certain cereal crop diseases by means of aerial photography. Hilgardia 26(5), 223-286.
Datt, B., Apan, A., Kelly, R., 2006. Early detection of exotic pests and diseases in Asian vegetables by imaging spectroscopy. Rural Industrial Research and Development Corporation (RIRDC) Publication (05/170).
Dutta, S., Bhattacharya, B.K., Rajak, D.R., Chattopadhyay, C., Dadhwal, V.K., Patel, N.K., Verma, R.S., 2008. Modelling regional level spatial distribution of aphid (Lipaphis erysimi) growth in Indian mustard using satellite-based remote sensing data. International Journal of Pest Management 54(1), 51-62.
Gitelson, A.A., Merzlyak, M.N., Chivkunova, O.B., 2001. Optical properties and nondestructive estimation of anthocyanin content in plant leaves. Photochemistry and Photobiology 74(1), 38-45.
Haboudane, D., Miller, J.R., Tremblay, N., Zarco-Tejada, P.J., Dextraze, L., 2002. Integrated narrow-band vegetation indices for prediction of crop chlorophyll content for application to precision agriculture. Remote Sensing of Environment 81(2-3), 416-426.
Held, A.A., Jupp, D.L.B., 1995. Forest monitoring with high spectral/spatial remote sensing tools: Case studies.
Hong, S., Rim, S., Lee, J., Kim, J., 1997 (August). Remote sensing for estimating chlorophyll amount in rice canopies. In: IGARSS'97. 1997 IEEE International Geoscience and Remote Sensing Symposium Proceedings. Remote Sensing-A Scientific Vision for Sustainable Development 1, 89-91.
Jordan, C.F., 1969. Derivation of leaf‐area index from quality of light on the forest floor. Ecology 50(4), 663-666.
Kumar, J., Vashisth, A., Sehgal, V.K., Gupta, V.K., 2013. Assessment of aphid infestation in mustard by hyperspectral remote sensing. Journal of the Indian Society of Remote Sensing 41(1), 83-90.
Laudien, R., 2005. Entwicklung eines GIS-gestützten schlagbezogenen ührungsinformationssystems für die Zuckerwirtschaft (Development of a field-and GIS-based management information system for the sugar beet industry). University of Hohenheim.
Niño, E., 2002. The use of a multispectral radiometer to detect greenbug, Schizaphis graminum (Rodani) damage in winter wheat, Triticum aestivum L. Doctoral dissertation, West Texas A&M University.
Nutter, W.F., Litterell, R.H., 1996. Relationship between defoliation canopy reflectance and pod yield in the peanut late leaf spot pathosystem. Crop Protection 15, 135-142.
Oerke, E.C., Dehne, H.W., 2004. Safeguarding production-losses in major crops and the role of crop protection. Crop Protection 23(4), 275-285.
Ou, S.H., 1985. Rice Diseases. Commonwealth Mycological Institute, Kew, Surrey, England. pp. 109-200.
Peñuelas, J., Filella, I., Gamon, J.A., 1995. Assessment of photosynthetic radiation‐use efficiency with spectral reflectance. New Phytologist 131(3), 291-296.
Peñuelas, J., Gamon, J.A., Fredeen, A.L., Merino, J., Field, C.B., 1994. Physiological Changes in Nitrogen-and water-limited sunflower leaves. Remote Sensing of Environment 48(2), 135-146.
Riedell, W.E., Blackmer, T.M., 1999. Leaf reflectance spectra of cereal aphid‐damaged wheat. Crop Science 39(6), 1835-1840.
Rondeaux, G., Steven, M., Baret, F., 1996. Optimization of soil-adjusted vegetation indices. Remote Sensing of Environment 55(2), 95-107.
Rousel, J.W., Haas, R.H., Schell, J.A., Deering, D.W., 1973. Monitoring vegetation systems in the Great Plains with ERTS. In: Proceedings of the Third Earth Resources Technology Satellite-1 Symposium; NASA SP-351. pp. 309-317.
Sampson, P.H., Mohammed, G.H., Colombo, S.J., Noland, T.L., Miller, J.R., Zarco-Tejada, P.J., 1998. Bioindicators of Forest Sustainability Progress Report. Ontario Forest Research Institute. Forest Research Information Paper (142), 18.
Schmitz, R.F., Gibson, K.E., 1996. Douglas-fir Beetle. USDA For. Serv. Forest Insect and Disease Leaflet (FIDL). 5. R1-96-87. Washington, DC.
Shibayama, M., Akiyama, T., 1989. Seasonal visible, near-infrared and mid-infrared spectra of rice canopies in relation to LAI and above-ground dry phytomass. Remote Sensing of Environment 27(2), 119-127.
Shibayama, M., Takahashi, W., Morinaga, S., Akiyama, T., 1993. Canopy water deficit detection in paddy rice using a high resolution field spectroradiometer. Remote Sensing of Environment 45(2), 117-126.
Taubenhaus, J.J., Ezekiel, W.N., Neblette, C.B., 1929. Aeroplane photography in the study of cotton root rot. Phytopathology 19(6), 1025-1029.
Thomson, A.J., Goodenough, D.G., Barclay, H.J., Lee, Y.J., Sturrock, R.N., 1996. Effects of laminated root rot (Phellinus weirii) on Douglas-fir foliar chemistry. Canadian Journal of Forest Research 26(8), 1440-1445.
Toler, R.W., Smith, B.D., Harlan, J.C., 1981. Use of aerial color infrared photography to evaluate crop disease. Plant Diseases 65(1), 24-31.
Trotter, G.M., Whitehead, D., Pinkney, E.J., 2002. The photochemical reflectance index as a measure of photosynthetic light use efficiency for plants with varying foliar nitrogen contents. International Journal of Remote Sensing 23(6), 1207-1212.
Tucker, C.J., Holben, B.N., Elgin Jr, J.H., McMurtrey III, J.E., 1981. Remote sensing of total dry-matter accumulation in winter wheat. Remote Sensing of Environment 11, 171-189.
Underwood, E., Ustin, S., DiPietro, D., 2003. Mapping non-native plants using hyperspectral imagery. Remote Sensing of Environment 86(2), 150-161.
Vigier, B.J., Pattey, E., Strachan, I.B., 2004. Narrowband vegetation indexes and detection of disease damage in soybeans. IEEE Geoscience and Remote Sensing Letters 1(4), 255-259.
Yamamoto, H., Suzuki, Y., Iwano, M., Hayakawa, S., 1995. Remote sensing of occurrence place of rice blast disease by infrared thermal image. Japanese Journal of Crop Science 64(3), 467-474.
Yang, Z., Rao, M.N., Elliott, N.C., Kindler, D., Popham, T.W., 2005. Using ground based multispectral radiometry to detect stress in wheat caused by greenbug (Homoptera: Aphididae) infestation. Computers and Electronics in Agriculture 47(2), 121-135.
Zarco-Tejada, P.J., Miller, J.R., Noland, T.L., Mohammed, G.H., Sampson, P.H., 2001. Scaling-up and model inversion methods with narrowband optical indices for chlorophyll content estimation in closed forest canopies with hyperspectral data. IEEE Transactions on Geoscience and Remote Sensing 39(7), 1491-1507.
Zhang, M., Qin, Z., Liu, X., Ustin, S.L., 2003. Detection of stress in tomatoes induced by late blight disease in California, USA, using hyperspectral remote sensing. International Journal of Applied Earth Observation and Geoinformation 4(4), 295-310.