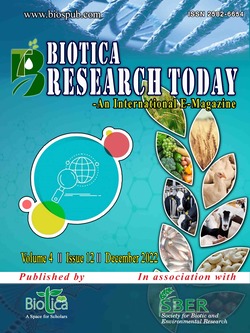
Artificial Intelligence (AI) Applications in Plant Parasitic Nematode Detection and Identification
Prabhu, S.*
Dept. of Plant Nematology, Tamil Nadu Agricultural University, Coimbatore, Tamil Nadu (641 003), India
R. Poorniammal
Dept. of Agricultural Microbiology, Tamil Nadu Agricultural University, Coimbatore, Tamil Nadu (641 003), India
DOI: NIL
Keywords: Artificial intelligence, Identification, Machine learning, Nematode
Abstract
Pest prediction techniques help treat various plant pest and diseases. Prediction and downstream prevention require knowledge of pest etiology, which is common in some symptoms and infection, such as those caused by soil-borne organisms can pose a challenge. Fortunately, the advent of machine learning tools has enabled researchers to unravel the intricate interactions between hosts and infections without relying on difficult assumptions. Recently, the application of artificial intelligence (AI) in agriculture is of crucial importance. The introduction of technology in agriculture can be approached creatively. Controlling nematode infections in crops during the growth phase is of crucial importance. Early identification, categorization and analysis of nematode infections and potential remedial actions are always beneficial to agricultural progress. The identification and categorization of nematodes in crops, especially fruits, vegetables and floriculture, are critical for proper nematode management.
Downloads
not found
Reference
Akintayo, A., Tylka, G.L., Singh, A.K., Ganapathysubramanian, B., Singh, A., Sarkar, S., 2018. A deep learning framework to discern and count microscopic nematode eggs. Scientific Reports 8, 1-11.
Hakim, A., Mor, Y., Toker, I.A., Levine, A., Neuhof, M., Markovitz, Y., Rechavi, O., 2018. WorMachine: Machine learning-based phenotypic analysis tool for worms. BMC Biology 16, 1-11.
Kumar, V., Khan, M.R., Walia, R.K., 2020. Crop Loss Estimations due to Plant-Parasitic Nematodes in Major Crops in India. Natl. Acad. Sci. Lett. 43, 409-412. DOI: https://doi.org/10.1007/s40009-020-00895-2.
Uhlemann, J., Cawley, O., Kakouli-Duarte, T., 2020. Nematode Identification using Artificial Neural Networks. In: Proceedings of the 1st International Conference on Deep Learning Theory and Applications-DeLTA, Paris, France, 8-10 July, pp. 13-22.