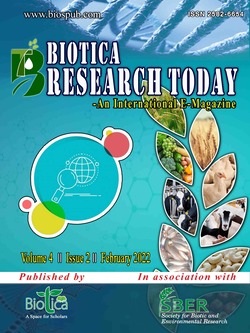
Generalized Least Square Method for Regression Analysis
Vaibhav Chittora
Dr. Y.S. Parmar University of Horticulture and Forestry, Nauni, Solan, Himachal Pradesh (173 230), India
DOI: NIL
Keywords: GLS, OLS, Variance matrix
Abstract
In regression analysis while estimating the parameters our data should follow the assumption of classical regression to have the valid estimator of the regression parameters. Sometime while estimating the parameter data does not follow the assumption of classical regression like constant variance of residual and autocorrelation of residual in that situation classical approach of estimating the regression parameter ordinary least square will not give valid estimators in that situation generalized least square will used.
Downloads
not found
Reference
Greene, W.H., 2003. Econometric Analysis. Prentice Hall, New Jersey, p. 828.
Gujarati, D.N., 2005. Basic Econometrics. McGraw-Hill, New York, p. 947.
Montgomery, D.C., Peck, E., Vining, G.G., 2014. Introduction to Linear Regression Analysis. WILEY Singapore, p. 672.
Musau, M.V., Waititu, A.G.P., Wanjoya, A.K., 2015. Modelling Panel Data: Comparison of GLS Estimation and Robust Covariance Matrix Estimation. American Journal of Theoretical and Applied Statistics 4(3), 185-191.