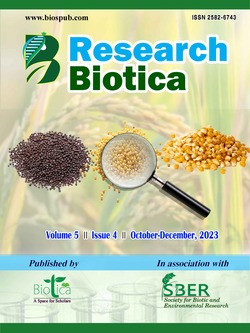
Role of Artificial Intelligence in Crop Protection
M. Pratheepa*
ICAR-National Bureau of Agricultural Insect Resources, Bengaluru, Karnataka (560 024), India
K. Subaharan
ICAR-National Bureau of Agricultural Insect Resources, Bengaluru, Karnataka (560 024), India
Richa Varshney
ICAR-National Bureau of Agricultural Insect Resources, Bengaluru, Karnataka (560 024), India
T. Venkatesan
ICAR-National Bureau of Agricultural Insect Resources, Bengaluru, Karnataka (560 024), India
S.N. Sushil
ICAR-National Bureau of Agricultural Insect Resources, Bengaluru, Karnataka (560 024), India
DOI: https://doi.org/10.54083/ResBio/5.4.2023/132-138
Keywords: Agriculture, Artificial intelligence, Crop protection, Integrated pest management, Insect detection, Mobile application
Abstract
In the era of 21st century, agriculture is facing many challenges now-a-days to feed the world population. The population growth is increasing day by day and it expected to cross 10 billion by 2050. Agriculture farming plays significant role in growth of Indian economy. India stands second in farm production all over the world. After the green revolution, India face production loss with an estimate of US$ 36 billion. The agriculture production decreases mainly because of insect pests, diseases and weeds in important agricultural crops. Hence, there is a need of transition in farming system to adopt advanced and innovative technologies for more and sustainable production. In recent years Artificial intelligence gained popularity in agriculture and provides solutions in several areas like big data analysis, pest and disease forewarning models, mobile applications in IPM, Information and ICT based crop-advisory system, insect detection, pest and disease identification, etc. In the proposed paper, AI based applications discussed in detail to provide insights into innovative technologies and pave the way for knowledge dissemination and adoption of AI based technologies for more effective crop production and protection.
Downloads
not found
Reference
Anonymous, 2023. Uzhavan - Bi-lingual mobile application. In: Google Play Store. Available at: https://play.google.com/store/apps/details?id=agri.tnagri&hl=en&gl=US. Accessed on: August 05, 2023.
Barbedo, J.G.A., 2019. Plant disease identification from individual lesions and spots using deep learning. Biosystems Engineering 180, 96-107. DOI: https://doi.org/10.1016/j.biosystemseng.2019.02.002.
Dhaliwal, G.S., Jindal, V., Mohindru, B., 2015. Crop losses due to insect pests: Global and Indian scenario. Indian Journal of Entomology 77(2), 165-168. DOI: https://doi.org/10.5958/0974-8172.2015.00033.4.
IUCN, 2011. The nature of progress: Annual report 2010. In: IUCN (website). The World Conservation Union, 2010. Available at: https://www.iucn.org/content/nature-progress-annual-report-2010. Accessed on: December 12, 2022.
Fedor, P., Vaňhara, J., Havel, J., Malenovský, I., Spellerberg, I., 2009. Artificial intelligence in pest insect monitoring. Systematic Entomology 34(2), 398-400. DOI: https://doi.org/10.1111/j.1365-3113.2008.00461.x.
Fedor, P., Peña-Méndez, E.M., Kucharczyk, H., Vanhara, J., Havel, J., Doricova, M., Prokop, P., 2014. Artificial neural networks in online semiautomated pest discriminability: An applied case with 2 Thrips species. Turkish Journal of Agriculture and Forestry 38(1), 111-124. DOI: https://doi.org/10.3906/tar-1305-8.
Ganesan, V., 2007. Decision Support System “Crop-9-DSS" for identified crops. World Academy of Science, Engineering and Technology: International Journal of Computer and Systems Engineering 1(12), 186-188.
Gassoumi, H., Prasad, N.R., Ellington, J.J., 2000. Neural network-based approach for insect classification in cotton ecosystems. In: Proceedings of International Conference on Intelligent Technologies, Bangkok, Thailand, December 13-15, 2000. pp. 1-7.
Guiam, A.C., Bawagan III, J.M.J., 2017. Insectify: An android application for digital insect identification using a convolutional neural network. CMSC 190 Special Problem, Institute of Computer Science. ICS University of the Philippines, Los Baños Laguna, Philippines. pp. 1-10.
Hassan, S.N.A., Rahman, N.S.A., Htike, Z.Z., Win, S.L., 2014. Automatic classification of insects using color-based and shape-based descriptors. International Journal of Applied Control, Electrical and Electronics Engineering (IJACEEE) 2(2), 23-35. URL: http://irep.iium.edu.my/id/eprint/38133.
Hu, Z., Liu, B., Zhao, Y., 2019. Agricultural Robot for Intelligent Detection of Pyralidae Insects. In: Agricultural Robots - Fundamentals and Applications. (Eds.) Zhou, J. and Zhang, B. IntechOpen. p. 144. DOI: https://doi.org/10.5772/intechopen.79460.
Kavi Kumar, K.S., Parikh, J., 1998. Climate change impacts on Indian agriculture: The Ricardinan approach. In: Measuring the Impact of Climate Change on Indian Agriculture. (Eds.) Dinar, A., Mendelsohn, R., Evenson, R., Parikh, J., Sanghi, A., Kavi Kumar, K.S., McKinsey, J. and Lonergen, S. World Bank Technical Paper No. 402, World Bank, Washington DC. pp. 141-183.
Mithra, V.S.S., Somasundaram, K., 2008. A model to simulate sweet potato growth. World Applied Sciences Journal 4(4), 568-577.
Mohanty, S.P., Hughes, D.P., Salathé, M., 2016. Using deep learning for image-based plant disease detection. Frontiers in Plant Science 7, 01419. DOI: https://doi.org/10.3389/fpls.2016.01419.
Nigam, S., Jain, R., Marwaha, S., Arora, A., Haque, M.A., Dheeraj, A., Singh, V.K., 2023. Deep transfer learning model for disease identification in wheat crop. Ecological Informatics 75, 102068. DOI: https://doi.org/10.1016/j.ecoinf.2023.102068.
Ngugi, L.C., Abelwahab, M., Abo-Zahhad, M., 2020. Recent advances in image processing techniques for automated leaf pest and disease recognition - A review. Information Processing in Agriculture 8(1), 27-51. DOI: https://doi.org/10.1016/j.inpa.2020.04.004.
Pratheepa, M., Subaharan, K., Varshney, R., Nagesh, M., 2022. Mobile Application on BIPM ON TUTA. In: Souvenir-cum-compendium of Abstracts: “Recent Advances in Agricultural, Biological and Applied Sciences Research”, Biotic Science Congress, 2022. 8th-9th August, 2022, Nagaon, Assam, India. Organized by Society for Biotic & Environmental Research (SBER), Tripura. p. 87.
Sehgal, J.L., Mandal, D.K., Mandal, C., Vadivelu, S., 1992. Agro-ecological regions of India. 2nd Edition, ICAR-National Bureau of Soil Survey & Land Use Planning, Nagpur. OXFORO & IBH Publishing Co. Pvt. Ltd. p. 130.
Selvaraj, M.G., Vergara, A., Ruiz, H., Safari, N., Elayabalan, S., Ocimati, W., Blomme, G., 2019. AI-powered banana diseases and pest detection. Plant Methods 15, 92. DOI: https://doi.org/10.1186/s13007-019-0475-z.
Silveira, M., Monteiroc, A., 2009. Automatic recognition and measurement of butterfly eyespot patterns. Biosystems 95(2), 130-136. DOI: https://doi.org/10.1016/j.biosystems.2008.09.004.
Singh, K.U., Kumar, A., Raja, L., Kumar, V., Kushwaha, A.K.S., Vashney, N., Chhetri, M., 2022. An artificial neural network-based pest identification and control in smart agriculture using wireless sensor networks. Journal of Food Quality 2022, 5801206. DOI: https://doi.org/10.1155/2022/5801206.
Thenmozhi, K., Reddy, U.S., 2017. Image processing techniques for insect shape detection in field crops. In: International Conference on Inventive Computing and Informatics. ICICI, 2017, Coimbatore, India. pp. 699-704. DOI: https://doi.org/10.1109/ICICI.2017.8365226.
Thenmozhi, K., Reddy, U.S., 2019. Crop pest classification based on deep convolutional neural network and transfer learning. Computers and Electronics in Agriculture 164, 104906. DOI: https://doi.org/10.1016/j.compag.2019.104906.
Valan, M., Makonyi, K., Maki, A., Vondráček, D., Ronquist, F., 2019. Automated taxonomic identification of insects with expert-level accuracy using effective feature transfer from convolutional networks. Systematic Biology 68(6), 876-895. DOI: https://doi.org/10.1093/sysbio/syz014.
Wang, J., Lin, C., Ji, L., Liang, A., 2012. A new automatic identification system of insect images at the order level. Knowledge-Based Systems 33, 102-110. DOI: https://doi.org/10.1016/j.knosys.2012.03.014.
Xia, D., Chen, P., Wang, B., Zhang, J., Xie, C., 2018. Insect detection and classification based on an improved convolutional neural network. Sensors 18(12), 4169. DOI: https://doi.org/10.3390/s18124169.